[ad_1]
There’s an age-old take relating to Apple and scorching new applied sciences: if the corporate hasn’t shipped no matter everyone else within the trade is at the moment specializing in, it should be behind.
That is not often the reality.
Apple’s enterprise is just like the proverbial iceberg: we solely see the tip of what the corporate’s doing, whereas the overwhelming majority of its analysis and growth efforts are looming beneath the floor. Simply take a look at its funds in its most up-to-date quarter: it spent $7.7 billion on R&D, accounting for greater than half of all of its working bills.
The most recent expertise to characteristic on this storyline is, after all, synthetic intelligence. How can the corporate compete on this burgeoning new market if it doesn’t come out with a chatbot or picture generator submit haste? (By no means thoughts that it nonetheless hasn’t shipped its digital actuality headset–that was the final market the place the corporate was clearly falling behind.)
However, as is all the time the case with this specific canard, the reality is that Apple’s been doing AI in its personal specific approach, and it’s not about chasing the market.
Get your studying on
One cause that Apple’s AI work is usually neglected is solely one in all terminology. Whereas the corporate doesn’t typically discuss “synthetic intelligence,” it does spend plenty of time discussing “machine studying” (ML), which is a vital underpinning to plenty of Apple’s newest applied sciences.
Although machine studying could technically solely be a subset of synthetic intelligence (and there’s some disagreement on even that) the 2 phrases are sometimes used interchangeably, no less than on a colloquial foundation. The big language fashions behind instruments like ChatGPT, Google’s Bard, and Microsoft’s new Bing chatbot make the most of machine studying applied sciences, as do picture turbines like DALL-E and Steady Diffusion. Broadly talking these are all applied sciences that contain algorithms that use knowledge to be taught and enhance.
Apple’s funding in machine studying is evident: in 2018 the corporate employed Google’s head of machine intelligence, John Giannandrea, as its Senior Vice President of Machine Studying and AI Technique, reporting on to Tim Prepare dinner. It additionally runs a uncommon public-facing web site the place it publishes a considerable amount of its machine-learning analysis and actively sponsors Ph.D. fellowships, recruits interns, and provides residencies. And it contributes to open-source machine studying tasks, for instance, optimizing the Steady Diffusion software program to run on its {hardware}.
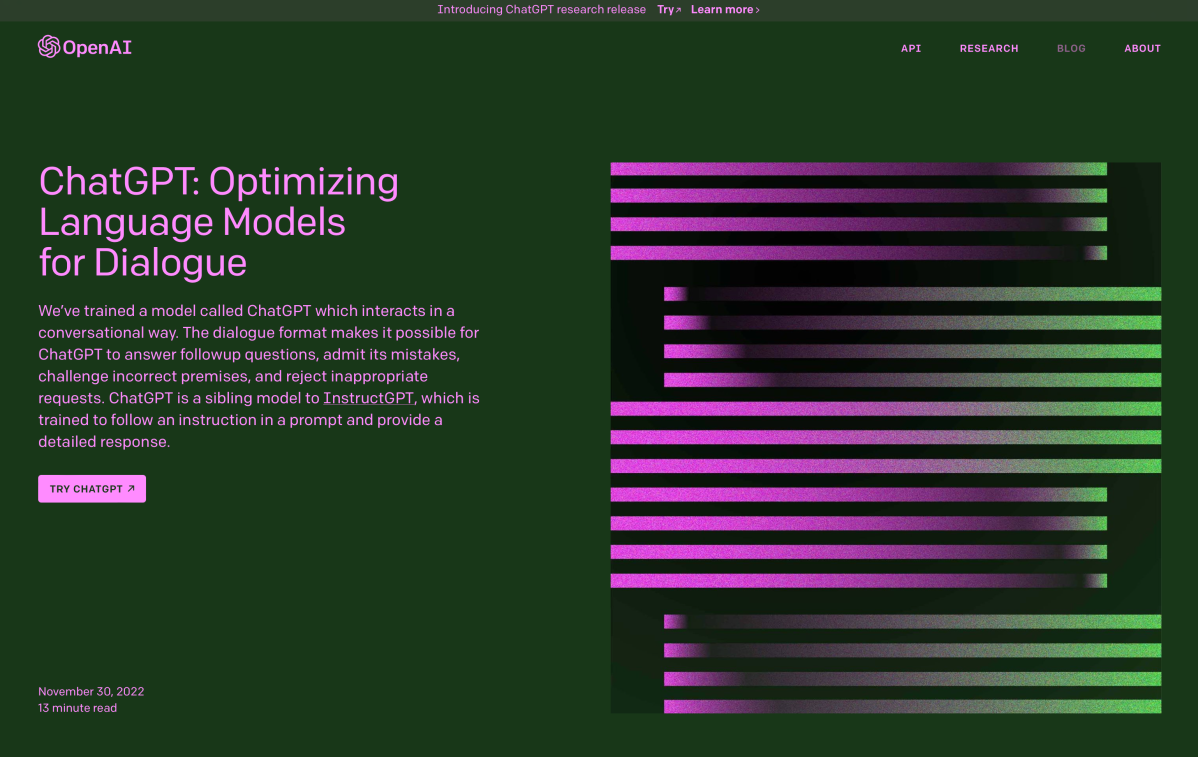
IDF
The cores of machine studying
You may concede that Apple is in ML, however maybe you’ll double down on asking what it has really produced with all of this funding.
A lot. In the event you’re a fan of options like Stay Textual content—the characteristic that lets you choose any textual content out of pictures or video—or the power to look your Pictures library for the phrase “canine” and really see all the images of canine you’ve taken, or the beta Stay Captions characteristic that may subtitle any video or audio taking part in out of your system, you’re benefiting from Apple’s machine studying analysis.
The corporate’s additionally created a complete framework known as CoreML to make it simple for builders to combine machine studying into their merchandise, and if that’s not sufficient, then keep in mind that each Apple-made processor courting again to 2017’s A11 Bionic has featured a devoted Neural Engine, optimized for operating machine studying algorithms—in the newest iteration, which options 16 cores, it might run an astounding 17 trillion operations per second, permitting, in typical Apple trend, machine studying fashions to run privately in your system as an alternative of counting on a cloud service.
If Apple’s spending the cash to commit a good portion of its processors to machine studying, then it’s most definitely placing its cash the place its mouth is.
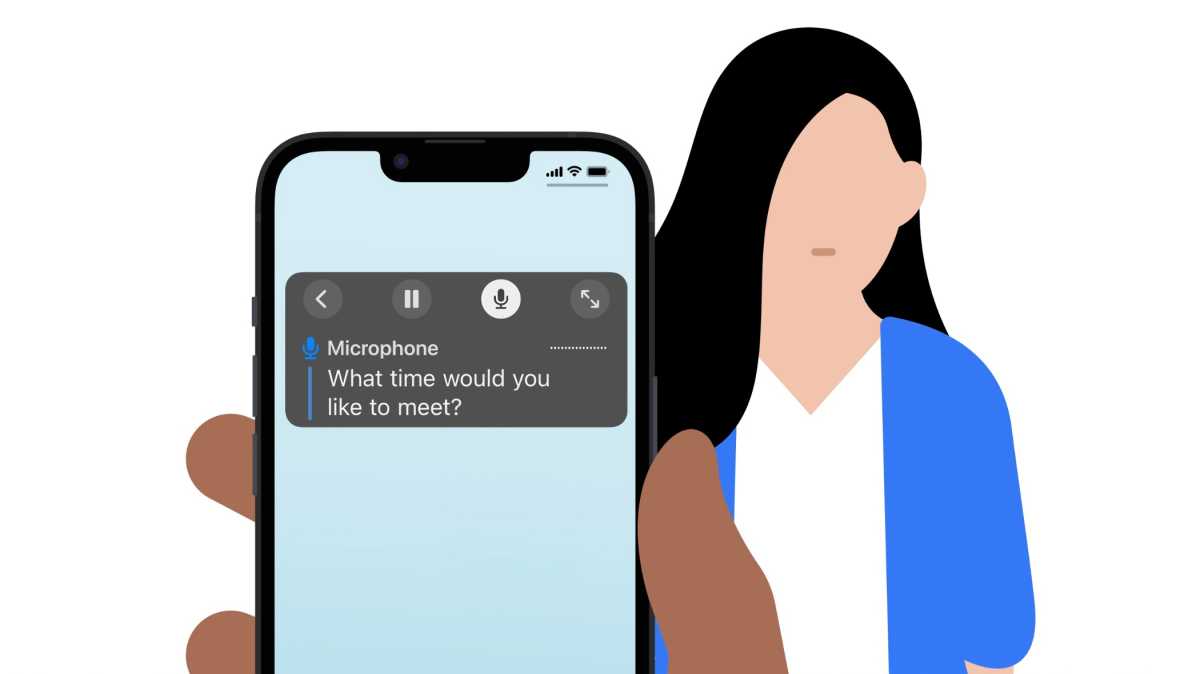
The Stay Captions beta is an instance of Apple’s analysis into machine studying.
Apple
Tip of the iceberg
Are there extra alternatives for Apple to extend its machine-learning footprint? Actually. The glib reply is that Siri’s typically lackluster efficiency could possibly be enhanced by the form of AI you see in current chatbots—although, given the really weird nature of a number of the conversations with Microsoft’s current foray, it appears seemingly Apple isn’t going to instantly bounce into the deep (studying) finish of the pool.
However the capability for these forms of programs to retain context and talk in a extra fluid and human method does have benefits that might and will work their approach into Apple’s digital assistant, even when in a extra restricted and managed method.
Likewise, the speech-to-text capabilities of Stay Captions and Apple’s dictation programs could possibly be enhanced had been Apple to do a number of the identical optimizations it’s performed with Steady Diffusion on the very spectacular Whisper speech recognition framework.
Once more, these aren’t as flashy as what lots of Apple’s rivals are doing out there, however neither does Apple have to chase that performance in the identical approach. Google and Microsoft, for instance, are utilizing AI to duke it out in search, a market that Apple doesn’t actually play in (although I’d hardly be against Apple utilizing a number of the underlying expertise to enhance its personal on-device search capabilities).
Ultimately, Apple’s use of machine studying stays pushed extra by the concept of the way it can improve what customers do, quite than simply present for its personal sake. And whereas that won’t seize the creativeness in the identical approach, it could in the end have an even bigger affect on customers’ lives. Which, to my thoughts, places Apple forward within the sport, not behind.
[ad_2]